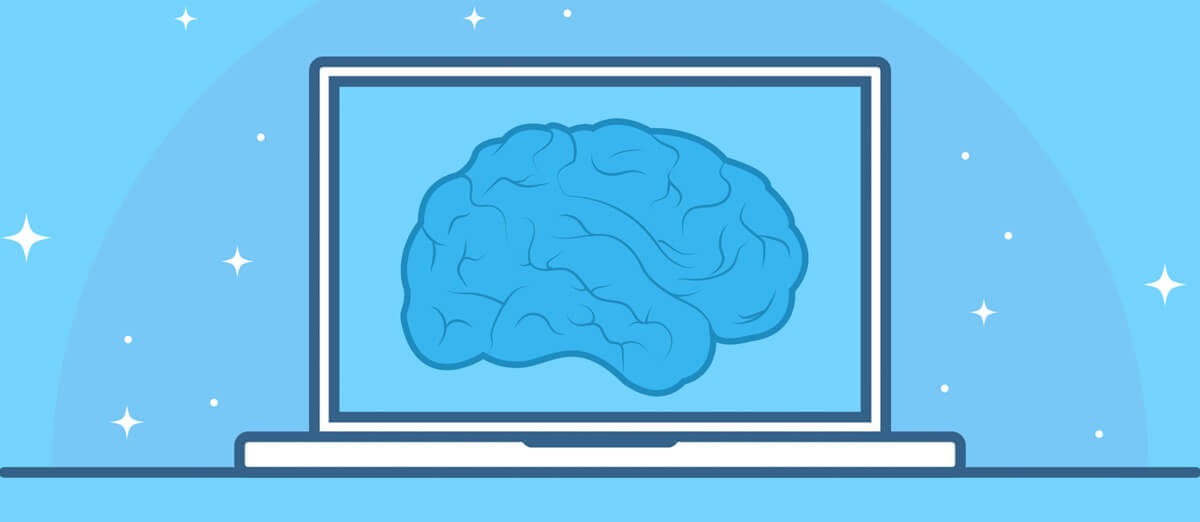
Having almost every facet of modern life connected through the internet confers various advantages, and one of the most significant is the ability to analyse performance data to an unprecedented extent. The more data you collect from myriad sources, the more accurately you can gauge business results: what tactics are working, and what needs to change.
Enterprise data science, however, takes this notion to its logical apogee. Instead of using data to rate particular methods, or even strategies, it uses it to rate entire businesses, producing holistic assessments that can easily lead to broad operational improvements.
This is something that the biggest businesses in the world have already invested heavily in pursuing, and with every passing month, it becomes cheaper and more accessible. But how is the enterprise data science world changing overall? Let’s look at 6 notable trends in this field:
Intuitive data visualization
Simply collating and interpreting data can be tricky for an enterprise-level business. Information from numerous departments brought together to be examined by various staff members, each with a different level of technical awareness. It can cause more confusion than anything else.
To temper this ever-increasing complexity without having to simplify it, companies are turning to intuitive visualization methods, forging patterns into easy-to-follow graphics and devising touch-friendly interfaces to manipulate them.
The effect is twofold: firstly, an enterprise with clearer internal analytics becomes better equipped to achieve improvements, and secondly, reporting performance results to non-technical clients becomes much easier.
Sophisticated forecasting
Using data to anticipate future requirements has long been a core role of data science, but recent developments have greatly expanded the possibilities. Today, a forward-thinking company can use sophisticated analysis to usefully forecast anything from how a particular operational change might affect sales to how much stock it will need during the winter.
The better the forecasting, the more money gets saved, and the easier the everyday running of a business becomes. It’s all about gathering distinct elements of a system together (something that SlashDB’s silo accessibility can bring to the table).
Rising security standards
It’s now been over a year since GDPR was implemented in the EU, and there’s been a huge rise in popular awareness of security concerns. People are more reluctant than ever before to trust businesses with their private data — yes, circumstances may often require them to, but that doesn’t mean that they’re happy about it.
Quelling this discontent requires an appreciation of how egregiously data science can be abused, and enterprise data science must reasonably encompass the vital matter of how a given enterprise justifies its analytics to its customer base.
If you’re running a large company and trying to advance your data science operations, be extremely careful with how you present your activities to those whose data you’re relying on. Brand reputation means a lot these days, and you don’t want to attract a social media firestorm by being overly casual about how you collect and use private data.
Natural language processing
Bridging the gap between raw data and trends suitable for human interpretation is a difficult process, even with the type of visualization that we already looked at — to reach a stronger level of data analysis, we need effective natural language processing (NLP), and that’s just what some of the biggest companies in the world have been working on.
Imagine the wide-reaching consequences of software being able to not only identify what’s being said but meaningfully parse it and relate it to overarching business goals. Sooner or later, there will come a time when someone can simply say “Give me a breakdown of predicted product profitability in the third quarter” and get a full automated forecast.
The rise of voice assistants has already shown what’s possible with present-day technology, and things like Google Duplex and Alibaba’s nascent offering are just over the horizon (though still very much in the testing phase). Keep an eye on the NLP industry, because it’s going to remain a core concern.
Enhanced personalization for more sales
Businesses of all types need to sell themselves, and whether they’re targeting high-end B2B clients or numerous B2C clients, personalization is the key to this. The more keenly you target a pitch, the more effective it will prove — and data science greatly enhances personalization.
Look at a scalable platform like NICE, designed to finely polish customer experience (CX) through the unification of smart modular applications. Every interaction with a customer factors into a cohesive assessment of their needs and preferences, all manifesting in CX delivery that automatically adapts over time to increase efficacy.
Extending this CX polish, the endgame of this personalization push is the optimal automation of entire sales pipelines. In the coming years, we’ll see numerous AI systems enter the game, using rich data to achieve results that no human could ever hope to rival through manual effort.
Hyper-efficient support systems
Automation can be applied to almost every part of a modern digital business, but something that’s particularly important for enterprise businesses is support infrastructure. Whether you sell products or offer services, you’re going to need to assist people with overcoming obstacles, understanding systems, and achieving goals.
A traditional enterprise business with 9-to-5 phone lines had a tough time, so the move to international 24/7 business hours (and the rise of alternative support channels) only made things tougher — at least, for a time. With the kind of data available to enterprise businesses now, they can viably implement much smarter support schemes.
For instance, a company can use a high-quality chatbot driven by analytics data to field most low-level requests and seamlessly escalate anything serious (here’s a range of chatbots segmented by purpose). That way, it can maintain a useful presence at all hours without needing to hire a huge support team. If a big business doesn’t use its rich data to save time, money, and effort, why bother collecting it at all?